Data Valuation: How to stand out in data strategy
- Feb 21, 2020
- 5 min read
Updated: Mar 2, 2020
Often, we look to technology to solve our problems and in the process creating new ones. You will need to do a few things differently in order to stand out, and one of them is to understand the paradox of what is happening right now in Data. A decade ago, we did not have enough data, and now we have too much data. This changes things in a significant manner because we have somewhat of a completely different problem.
To highlight this, let me walk you through an example of the issue.
TripAdvisor does a great job of collating reviews and getting a lot of information, but when you read through the reviews of a potential place you might stay at. You often read many reviews but there is just one that really seals the deal for you and most likely in a negative way. A hundred glowing reviews can be undone by that one person saying there was a spider on the bed. When this happens do you consider the hundred positive reviews or that one review?
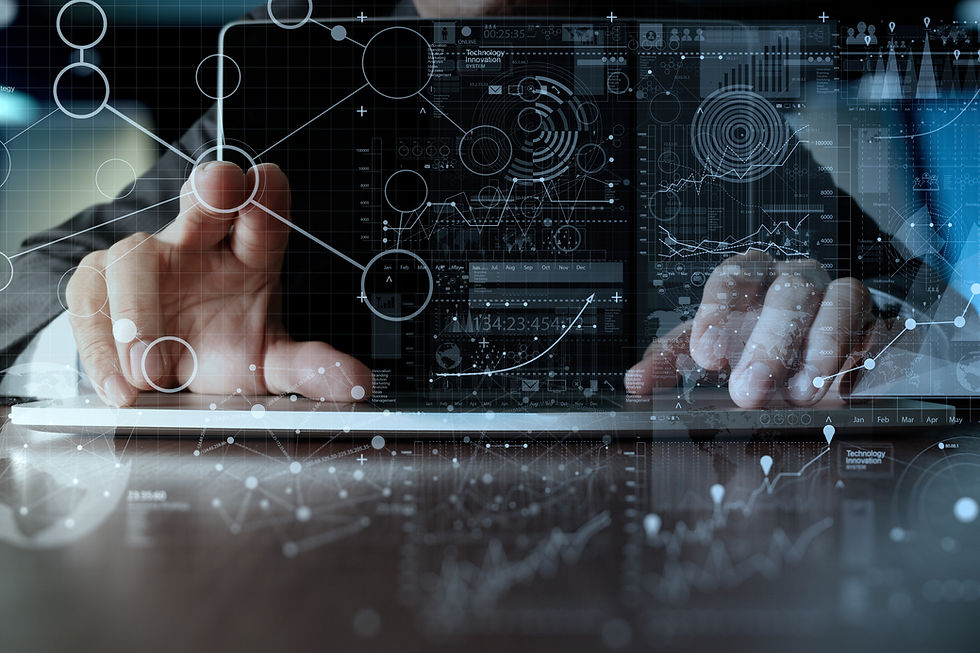
There was a survey done by the BBC a while back that asked the simple question, "What city is bigger Minneapolis or Detroit?". While this seems innocuous the results were interesting. When the question was asked in America 43% answered correctly, whereas when asked in Germany 80% answered correctly. At first glance, this seems counter-intuitive but it is really not. What is happening here is that Americans know quite a few facts about both cities which means they have more data points to go through, most Germans surveyed had not heard of Minneapolis but had heard of Detroit. The Germans used that one piece of knowledge to make their selection. After all, if you have heard of a city, you can imagine that it is most likely bigger than the ones you have not heard of, it's a simple straight forward deduction. Now take this example and look at how many data points you might be generating, and think through the relevance of what you are now looking at. Often it is one piece of data in all of the rest that is important and with the proliferation of data it is getting harder to figure out which one that is. Fortunately, the reality is that we are gearing up with AI and approaches that take additional levels of a neural net to figure this out because it is becoming impossible to quickly ascertain the correct intelligence without looking at all the things available. The key is to find the correct data in the data swamp you may have created. Data Management then really gets redefined through this because we supply that data and as we add more layers of orthogonal data (relevant but not required data) we need to be mindful of how it is being interpreted in the business layer. I will be devoting a substantial amount of time talking about how to get that value out of the data in following articles.
So where to start if you have a lot of data and not a lot of information? Let's go through steps:
1. Most likely you are leveraging Big Data in some way, lots of stored unstructured data and also some structured data. The big secret here is to look deeper, and by that, I mean you need to realize that all data has a seldom understood additional component. Underneath Structured and Unstructured data is yet another layer we can label as ‘repeating’ and ‘non repeating’ data. To expound on this a bit, let us look at two types of companies and the data they produce. First, we take the example of a Utility company, in recent years they have transformed using Smartmeters that transmit data through a mesh network to support usage and billing cycles. That data is sent once or twice a day and is considered ‘Unstructured, repeating data’. While they also have a lot of other data, this is the data of value. Let us look at a Media company, they do not have the same business but a lot of their data is in Video, email, texts and social media platforms. This data is clearly ‘Unstructured non-repeating’ data.
Now that we understand the different types of data, I would point out that the data architecture to support these is vastly different. The first, a large Hadoop install that can store and search large amounts of unstructured data is a good start. However, for the Media company not only do we need to store the data differently because we do not know when it is coming (real time component) we also need to understand it completely differently. This type of data can be especially complex because it is key that we understand complex linguistic nuances very quickly. An example would be looking at social media information during a product, or movie launch. Someone could say “Yeah! I really loved that movie” and depending on the tone he could be sarcastic or he could be positive. Which one is it? Essentially, we need to get to a semantic level of understanding and as Bill Inmon coined the phrase ‘Textual Disambiguation’. What he means is that things are ambiguous until you remove that from the text somehow with a good level of AI so that you can understand exactly what is meant. This applies to a lot of companies but the first step is to look at what and how you are getting that data. Unstructured repeating or Unstructured non-repeating. The examples above give you the idea of how to architect accordingly. Most companies we survey often have the wrong architecture and then are unable to really get any value from the implementation itself. This factored heavily into a massive drop off of Hadoop implementations in general as customers did not get the value they expected.
The second step is to look at data value. Everyone talks a great game about how your data is valuable but if you ask them to value that data set for instance, they won’t be able to do it. You need a valuation strategy to start understanding the value you are generating from your data sets. In a recent example we worked with a client that was focused on new customer acquisition for their hardware business. When we reviewed the data sets, we found that their renewable customer data set was more valuable and likely to generate more revenue. This was something they had not realized and they were able to increase profitability just by this insight alone. While there are diverse data valuation processes and strategies out there it is good to know the baseline approaches (such as treating data as a strategic asset) and be able to customize these to your needs. For instance, do you want to monetize data, understand the intrinsic value of the data or be worried about replacing that data if it is lost. Sometimes customers want to just look at data quality and completeness. Whichever view might you have, data valuation is likely something that most companies overlook.
To recap, you need to look carefully at both step 1 and step 2 to really begin to sort out the plethora of your data and bring some value to your data, this may result in changes to your architecture and changes to your process as well as looking at data curation. Curation is a term that is now being applied to data because there is a need for data to be ready, available and prepared for viewing when someone will actually need it, this is especially complex and deserves its own blog which will be the next one you will see.
ABOUT THE AUTHOR
Asim Razvi | VP, Data Management ONIS Solutions
@DataAsArt
Asim Razvi has been focused on Business Intelligence for the last 20 years, currently, as the head of the Data Strategy for Onis Consulting, he leads the analytics and data approach for strategic accounts. He brings a wide background across Media, Communications and Finance to Onis and has built Business Intelligence practices for PwC, Cognizant and Accenture. Asim was formerly the Head of Education and Research for TDWI where his focus was to rebuild the TDWI brand through alignment with Analytics, Big Data and Cloud thought leadership.
Comments